Across the natural world, cells organise themselves into a wonderful array of shapes and structures. But how do they do this? Plus, building bones, plant sex in space, and a rather plump gene of the month.
In this episode

00:58 - Which way is up?
Which way is up?
with Veronica Grieneisen, John innes Centre, Norwich
Kat - This month I'm reporting back from the Genetics Society Autumn Meeting, which took place at the Royal Society in London. Called "Genes to Shape", the talks brought together researchers ranging from mathematicians and physicists to developmental biologists to discuss how biological shapes are created.
Dr Veronica Grieneisen from the John Innes Centre in Norwich, is figuring out how cells know which way is up, and start to organise themselves into tissues.
Veronica - For a scientist, I think we look at nature and we have many questions. But what's really close to my heart is to try to understand how cells can understand position within themselves but also amongst themselves. And so, that's not only in plant cells, but also in animal cells which are moving around.
Kat - I guess how do we know where we are and what we're doing?
Veronica - Exactly and not only where we are and what we're doing, but what is our head and what is our tail. And so, for us, we already come with our head and with our feet, but for plants say, or for cells, they actually have to establish that information. I mean, if you think about a cell, you have a bag of proteins in the nucleus, but who's telling that cell what part of that membrane will be at the front and what part of that membrane will be at the back? And so, that is something that we're investigating and we see that this information is coming from within and it can be spontaneously generated.
Kat - I really loved it in your talk when you showed a blob which is a cell that had had its nucleus, its DNA, taken away and you kind of poke it, and it moves by itself. What's going on there?
Veronica - So, those are really classical experiments and it's quite mind boggling because then the cell without a nucleus, it's able to detect a gradient. It's able to do really complex things. What we realise there is that it's all through the dynamics of these proteins within. And so, that's what we're able to explain, only through modelling. So, we're bringing in mathematical formulations of what's happening and what's most interesting for us is now, we're discovering that exactly the same process is happening in plants while these two, animals and plants, they have diverged 1.6 billion years ago. So, this logic of how a cell polarises is really, really ancient.
Kat - And it's the same proteins - you have proteins at the front of the cells, proteins at the back, and they're the same proteins doing this.
Veronica - Basically, they're very similar. So, these are the small G proteins, but what is the same is the mechanism. So, maybe the proteins are a little bit different but the way they do it is the same. We think that afterwards, when multi-cellularity has been evolved, although it's been done independently in plants and animals, you're still stuck with the same principles.
Kat - And so ultimately, where do you hope that your work is going to take you because at the moment, you're just kind of modelling single cells. Where do you want to go with it?
Veronica - So, indeed. We're understanding the single cell, but what we're doing now is we're putting them together. So first, how does one cell speak to its neighbouring cell? So, how do you get interesting conversation between two cells? For example in plants, we know that we have these jigsaw puzzle-like shaped cells and they have to coordinate their jigsaw shape with their neighbouring cells. What we saw is that the way that they communicate can also be extended over many, many cells. So then, suddenly we can understand how our organs can give information from one region to the other. So basically, it's everything about scaling. We understand first the individual cell then its interactions, and then suddenly, we can understand more of this emerging behaviour on the level of many, many cells.
Kat - That was Veronica Grieneisen from the John Innes Centre in Norwich.

04:33 - Pollen tubes are go!
Pollen tubes are go!
with Anja Geitmann, University of Montreal
Kat - At the University of Montreal in Canada, Professor Anja Geitmann is developing models to explain how tiny tubes grow out of pollen grains to fertilise flowers under different conditions, such as zero gravity - her work has featured in the media with headlines such as "sex in space!" and worse. Naked Scientist Simon Bishop spoke to her to find out what she's actually up to.
Anja - We try to understand how plants function. Plants consists of cells just like the human body does. Only that the difference between the cells of a plant and that of a human is that the cells are surrounded by a wall and this wall consists, for example, of cellulose microfibrils. So, this is the stuff your clothes are also made from. And so, in order for such a cell to grow, it has to deform its cellulose material. In order to form a particular shape, it has to regulate the material properties, just like a balloon that blows up and becomes a sphere or becomes something more interesting. The plant cell that we work with is a very, very long tube. It's a catheter-like delivery tool of the sperm cells that serves to bring the sperm cells from the pollen grains to the ovules where the egg cells are housed in the flower. And so, in order to understand how this tube is formed, we do all kinds of stuff to it. We use inhibitors, we use enzymes, but the fun stuff we do is when we put it in the centrifuge and expose it to altered gravity conditions.
Simon - So, what do you find out by putting a plant cell, a pollen tube into first a microscope and then put it inside a centrifuge?
Anja - So, the centrifuge had the purpose to increase gravity, the effect of gravity. What we found is that all kinds of processes that are necessary in the cell to allow it to grow are affected. So, in the cell, things have to be transported from one end of the cell to the other. That is the same process that occurs for example in neurons, the cells of the human brain. This transfer process was affected strongly by gravity either by hypergravity or by microgravity - so, the absence of gravity. What we found is that as a result of this, growth was compromised and cell shape was altered. And from that, we can conclude that intracellular processes, although they occur at a micron scale are affected by mechanical influences such as increased gravity or lack of gravity.
Simon - So obviously, plants in natural conditions wouldn't experience extreme gravitational forces, but what you're doing is testing this to the limit so you can understand the forces that are normally involved in building a pollen tube.
Anja - So, the pollen tube is a crucial step in reproduction of plants that delivers the sperm cells. Without pollen tube growth, we won't have fruit, we won't have the next generation of plants. This process is easily affected by all kinds of influences. And so, we can imagine that effect such as microgravity or hypergravity - microgravity would be the case on the international space station or any other space travelling vessel, or hypergravity on a planet bigger than ours would affect plant growth. And so, we simply try to understand how plants function and use this as a tool - altered gravity as a tool to affect cellular functioning from a mechanical point of view.
Simon - And where next?
Anja - Okay, so the pollen tube is the cell that has very simple geometry. It's long and cylindrical. But what we really want to understand is how much more complex shapes are formed. So for example, if you look at the surface of a leaf, it has most intricate cellular shapes and we would like to understand how these complex shapes are formed and how they become to be functional during the development of a plant embryo into an adult plant.
Simon - Any plans to put other leaves and other plants into centrifuges?
Anja - That will be fun - putting an entire plant into a centrifuge. What has been done of course is that we have grown plants in space. That means microgravity conditions and plant functioning is affected by this process. It's very difficult to actually pull apart what is the effect of the absence of gravity and what is the effect for example of the absence of convection. Convection is the absence of air movement that is a result of the absence of gravity. And so, there's a whole lot of challenges that are out there for us.
Kat - That was Anja Geitmann from the University of Montreal. And now it's time for a roundup of this month's genetics news.

09:00 - Snake genomes slither into view
Snake genomes slither into view
In a pair of papers published in the journal PNAS, two international teams of researchers have described the first complete genome sequences of the Burmese python and the king cobra. Although snakes have broadly similar genomes to many other vertebrates, they've rapidly evolved some extreme traits, such as the production of highly toxic venom by cobras, or the ability to eat and digest their prey whole.
Pythons achieve this by ramping up their metabolism and increasing the mass of their internal organs in a matter of hours. The new python genome challenges the current idea that this ability is due to changes in genes themselves. Instead, it suggests that more subtle changes in gene activity, protein structure and the organisation of genes are at work. In addition to changes in individual genes, the scientists think the snakes' extreme characteristics could be linked to gains or losses of whole families of genes. And they found that snake genomes have been evolving at one of the fastest rates of any vertebrate.
The researchers think their studies will shed light on how other species, including our own, have evolved key characteristics. And they also suggest their findings could reveal insights into human health such as metabolic diseases, intestinal and stomach problems, Crohn's disease, organ failure and heart disease.
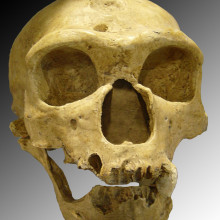
10:18 - Oldest Homo genome revealed
Oldest Homo genome revealed
Scientists from the Max Planck Institute for Evolutionary Anthropology in Germany have used new techniques to extract and analyse DNA from the bones of human ancestors from 400,000 years ago - the oldest early human samples ever analysed, publishing their findings in the journal Nature. The bones were recovered from a cave in northern Spain called Sima de los Huesos, or the Bone pit, are from the genus Homo, and are much older than our own species, Homo sapiens. Using just two grams of powdered bone, the researchers managed to extract and sequence the DNA from mitochondria, the 'energy factories' of the cells.
Analysis showed the DNA was similar to that taken from Denisovans, extinct relatives of Neandertals that lived around 700,000 years ago in Siberia. This is unexpected, as the bones look more similar to Neandertal remains. It may be that the Sima ancestors are related to an even older common ancestor of both Neanderthals and Denisovans, or that another group of mysterious hominins brought their genes over from Siberia.
The new results suggests that the origins of modern humans are more complex than previously thought, and the scientists are carrying out further analysis to try and straighten out the relationships between us and our early ancestors.

11:46 - Modelling flowers
Modelling flowers
with Jan Traas, ENS Lyon, France
Kat - Professor Jan Traas works at the ENS in Lyon, France. He and his team are using computer programmes to understand how flowers grow into their beautiful shapes. I asked him how he manages to turn the complexity of a natural structure like a flower into numbers that can be crunched.
Jan - Well, one of the major keywords is quantitative biology. If you want to use mathematical approaches, modelling approaches, you really need quantified data to go beyond qualitative conclusions, like it's bigger or it's smaller. You would like to know, you want to know how much bigger, how much smaller. That doesn't only include concentrations of proteins, sizes of cells, but also, what are the mechanical properties of these cells? What is the pressure the inside the cells? And this is really challenging.
Kat - So, you take all these measurements, these numbers. Presumably then you just stick them in a big computer and see what happens.
Jan - Well, that's what we originally wanted to do, but we realised very early on that well, modelling is actually a form of simplification. So, it's not just like sticking or putting a huge amount of data in a computer and then just push on the button. You really have to think about the processes in more abstract terms and you really have to think back how you want to simplify them. Simplification is really the keyword when you're doing modelling and when you're doing mathematical modelling. And that's of course extremely difficult to simplify complex things.
Kat - And then presumably, when you've got a mathematical model, you have to go and check that it actually does bear up with the real plants.
Jan - Exactly, yes. So, there's one thing we learned over the last years. I mean, for a while, biologists in our field which is molecular biology, cell biology, we'll tend into think in mainly qualitative terms as I've just said.
Kat - So, what something looks like, how it grows?
Jan - Yeah, just it was big or smaller, or it has changed, but you know, not really going into the details. When you're doing models that actually, all models are wrong and simplification also is necessarily going to be wrong. But it's because you can say where things are wrong in your model, that you can just go further and prove your model.
Kat - Presumably, making these models takes a huge amount of computing power as well.
Jan - Yes. Some of the models that we make currently, physical models in a form of virtual tissue, about a couple of hundred cells. To compute changes in shape can take up to 20 hours on a laptop computer. So, with just one simulation, you find out you didn't put in the right parameter of values. So, you start again, etc, etc. Well, that was really a problem, but our colleagues - computer scientists - they have apparently found other ways which can go from the initial 20 hours to about 5 minutes. These are computer programmes that are also used to train surgeons for instance where they can do sort of in silico operations and see what the effects are like you're pulling on this organ and you see effects on other organs. So, these are called live modelling methods that can also be applied to plants.
Kat - So, in your talk, you showed basically kind of small buds and just little buds growing out. Is it your dream one day to have a model that you can grow an entire flower?
Jan - Yes. Well, that definitely - the long term aim is really to have a virtual flower and to have as much information in there and hypothesis in there that we can. We'll have a flower. We'll also try to couple this model of a 3-dimensional tissue with other models that are at the level of the whole plant, trees, etc. So, the future that I think we're speaking in about 10, 20 years would be to couple all these models from molecular networks to the entire plant or animal, and to be able to go across the scales with these models. But a single model will not be possible, I think.
Kat - Is there a particular flower in mind? Do you have a favourite one that you would particularly like to see in the computer?
Jan - Well, there's many flowers in the computer. I think that, you know, so far our favourite flower is Arabidopsis, but it's not a very interesting one in terms of beauty of the structure. In our laboratory, we're working on some other types of flowers, particularly roses. I think we're also working on petunia for instance which can be beautiful flowers with very complex shapes. I think well, for me, that would be very good to have models running off of one of these flowers - roses and petunias.
Kat - That was Professor Jan Traas from ENS in Lyon.
17:23 - Building bones
Building bones
with James Sharpe, Centre for Genomic Regulation, Barcelona
Kat - Moving from plants to animals, Professor James Sharpe, at the Centre for Genomic Regulation in Barcelona, Spain is using mathematical models to understand how vertebrates like mammals and birds build a skeleton. I asked him to explain how he and his team start to model something as complex as this.
James - So, it probably is a complex thing. In our case, we're trying to address the very first basic steps that have to be taken so that it's not too complicated. It's a question of knowing how many bones to have in each position in your structure. So for example for the arm, we have one bone in the upper arm, we have two bones, the ulna and radius, in the lower arm, and then we have five fingers. Our research is to try and understand how that basic arrangement is laid out.
Kat - Now, there's an existing model of how this works and that you have a gradient of a chemical that goes across the developing limb. It goes from one side to the other and that tells you where the fingers go. But you're coming at this from a different angle. What's that?
James - So, we're actually trying to resolve the fact that there have been two competing ideas to explain this. The alternative idea to the one you mentioned was an idea that was first proposed by Alan Turing in 1952, although he didn't propose it specifically for limb development. It was a general observation about how cells might be able to organise themselves. But his theory which was a self-organising theory rather than a positional information theory has not been considered so relevant for this question over most of the decades of research in this field. We and others are starting to realise and do research to show that in fact, this self-organising idea of his is probably the main mechanism underlying the initial distribution of fingers in your hand for example.
Kat - So, in its purest form, how does Turing's idea work? What sort of patterns does it generate?
James - So, it always generates patterns that are periodic, which is to say they're alternating states...
Kat - Stripy...
James - ...stripy or spotty. In two dimensions, that's stripy or spotty. It can only do that because it does not have information about what's going on over the whole field of cells. It works purely by a local communication between neighbouring cells. For this reason, it can only produce stripy or spotty patterns. But this is exactly the kind of pattern that our fingers are - it's essentially a collection of five stripes next to each other.
Kat - So, if you just leave cells to do their own thing, more or less, within certain parameters, they will just organise themselves into stripes and you had a lovely quote in your talk about how Turing said that explains the stripes of a zebra, but not the horse bit. How is your work trying to understand the horse bit skeleton underneath?
James - So, in this case, as I say, the horse bit is the skeleton, but it is essentially just another example of a set of stripes. So, it is exact theory, unchanged, can be equally applied to do something like the fingers as to the stripes on the outside of a zebra. So, we're simply doing computer simulations, modelling and experiments in the lab to actually test whether his hypothesis is a reasonable explanation of that process.
Kat - But the sort of patterns that Turing's mathematics generates, they're not the straight, regulated digits that we see on a hand - they're kind of a bit blobby and a bit funny. What else is going on in the development of an actual hand to give that more regular pattern?
James - So this is where we realised that to actually produce something useful like a hand, rather than just a random Turing pattern, we actually needed collaboration between a Turing-type self-organising system, and also we need some kind of positional constraint that control this system, which could loosely be considered as a kind of positional information. So our general conclusion about how these systems work is that actually, rather than being a conflict between two alternative possible theories, both of these theories are equally necessary to explain the final result.
Kat - So we already know that there are lots and lots of different genes that are turned on in the hands, in the limbs, in different places and at different times. So it's the interaction of those with the underlying pattern that's shaping our bodies, literally?
James - This is exactly what we think. We're still probably at early days and we have a lot further to go, but it is exactly those gene expression patterns, signalling pathways, gradients, which regionalise the tissue, which have different values in different places, which control the self-organising process.
Kat - In your talk you talked about the long bone of the arm, the two bones of the lower arm and then the five fingers. What about the wrist? There's loads of bones in there!
James - That's a very good question actually. It's been speculated that it might relate to something that was discussed in connection with Turing more explicitly, which is the difference between zebra stripes and leopard spots. So you can change a parameter of a Turing system to switch it from making stripes to making spots. In fact, the wrist bones are more like spots, and the other bones are like stripes. So it could well be that the parameter is tuned just in the wrist region to convert from stripes to spots.
Kat - So with the models you're generating, understanding the stripes, the spots, the parameters that they're tuned within - where ultimately do you want to take this work? To figure out how we can build a whole skeleton?
James - Well, in fact our interest is not to explain the skeleton of the rest of the embryo, but instead to focus on the limb and to bring in the other factors that are relevant for the limb. Our goal is to make a complete computer simulation or model of all the relevant important aspects of limb development. So we're dealing with the skeleton at the moment. We're also dealing with mechanical morphogenesis of the bud, we're also trying to understand the regionalisation - why the hand is different from the arm? And our real goal is to bring all of these together into a single computer simulation where we can then understand, potentially, the higher level more complicated aspect of how these different subsets, subsystems interact with each other to create the full organ. The real ultimate goal is to explain organogenesis as a whole.
Kat - Now we more commonly hear Turing's name mentioned with regard to his efforts in code-breaking and things like that. Many people don't know that he had these ideas about biological systems. Do you think more people should be aware of it?
James - Personally I obviously think more people should be aware of it. In fact, I went to the centenary meeting of Turing last year in Cambridge and even there I was shocked to discover that the vast majority of researchers that had come to the meeting were completely unaware of his contribution to this area of developmental biology, and in fact it was an area of mathematics that he contributed to. It spawned an entire new field of mathematics, and yet still - as you say - many people are not aware of his contribution.
Kat - And now, sixty years later, you're trying to bring it back?
James - Absolutely!
Kat - That was Professor James Sharpe from the Centre for Genomic Regulation in Barcelona.

Does eating GM food make you GM too?
Kat:: Now it's time to look at your genetics questions. Listener Michael Veiga says "I heard that the human body re-generates itself almost completely every 7 years or so. If the adage that "You are what you eat" applies and if one were to only eat genetically modified foods for a period of 7 years, would that person's body be considered to be genetically modified as well by the end of the 7 years and what would the health implications of that be?" To answer, here's Michael Regnier from the Wellcome Trust. Michael:: I think that's a great question, it's a question we had recently on the Wellcome Trust blog recently. Most of us have heard the mantra "you are what you eat", and it's natural to wonder if the genes that we consume and take into our bodies can interact with our genes, which after all are pretty fundamental to who we are and how we feel. But, of course, we eat genes all the time.
Every cell contains DNA, whether it's in a human being or a cow or a fish or a spud or an ear of wheat. So whatever you eat it's going to have a lot of DNA in it. Of course, we don't then take on the features of cows, fish, potatoes and wheat when we eat them, and that's because when we digest our food, our bodies break it down into its constituent parts, and we take what we need and we excrete the rest. And the same goes for genes.
Rather than taking in whole genes from our food, the DNA is broken down. It's like if you take a sentence and cut it up into all the individual letters then you can make new words and new sentences out of those letters, and that's what we do. We use those letters - billions of them - to copy the words and sentences of our existing genes whenever we need to make new cells.
On top of that, our cells have special enzymes that check the genes that are being copied in this way. Most errors are spotted and fixed, so even if a string of DNA that makes up a non-human gene got through our stomach and got taken up into our cells, the chances are that it would be found and dealt with by those sorts of mechanisms. So, whether it's a normal gene from a normal cow or normal vegetable, or a modified gene, the chances of it getting through and being taken up in our genome are incredibly small.
Given that human beings have been eating foreign genes since forever, without literally becoming what we ate, I'd say that proves our bodies are pretty well set up to prevent any kind of crossover, and genetically engineered genes are no different. Kat:: Thanks to Michael Regnier from the Wellcome Trust for that answer. And if you've got any questions about genes, DNA and genetics, just email them to me at genetics@thenakedscientists.com.
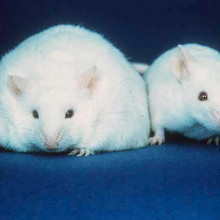
27:17 - Gene of the month - Tubby
Gene of the month - Tubby
with Kat Arney
Kat - And now, it's time for Gene of the Month and this one is a bit on the large side - it's called Tubby. The gene was first spotted in 1990 when researchers at the Jackson Laboratory in the US, a genetics research centre, noticed a strain of unusually fat mice in their breeding stocks - hence the name Tubby. Not only do animals with a mutation in the Tubby gene put on more weight, especially later in life, they also have progressive problems with their sight and hearing. But it's not just a quirk of mouse genetics - these symptoms closely mirror human genetic conditions such as Usher's, Bardet-Biedl and Alstrom's syndromes.
The Tubby gene itself was tracked down in 1996, and similar genes are found across a wide range of organisms, from animals to plants. But despite this ubiquity, relatively little is known about how the protein encoded by the Tubby gene, or related molecules, known as Tubby-like proteins, actually work. They seem to play a role in switching genes on, but also appear to play a part in sending signals inside cells. So, for now at least, Tubby is providing scientists with a plus-sized problem to get stuck into.
Comments
Add a comment