Will an artificially intelligent robot steal your job?
With the recent rise of the machines and robots - could an artificially intelligent robot take your job any time soon? And could they then take over the world, terminator-style? Join Graihagh Jackson as she journies into the world of cyborgs to see if Skynet, Ex Machina and the realms of science fiction could turn into science fact and if so, when? And what can we do about it...
In this episode
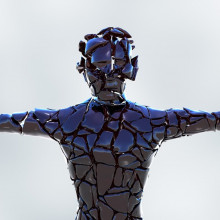
00:59 - The rise of the machines
The rise of the machines
with Professor Huw Price, University of Cambridge
Signs that the Artificial Intelligence industry is booming recently are everywhere. For instance, Google snatched DeepMind, London-based AI startup, from Facebook for a rumoured $400m and is using a computer named Watson to analyse our health records. But world-leading thinkers like Elon Musk and Stephen Hawking have expressed fear about the growing industry of artificial intelligence. But why? How could a machine be a threat to us? Graihagh Jackson sat down with Huw Price to discuss...
Huw - We can find particular historic figures who were very clear that at some point in the future machines would be capable of thinking and, of course, a great one is Alan Turing. Another one is a Cambridge trained mathematician and statistician called I.J. Good who grew up in the East End of London, won a scholarship to Cambridge, did a PhD with D.H. Hardy, a great Cambridge mathematician and then went to Bletchley Park to work with Turing. He and Turing used to spend their evenings off talking about the future of machine intelligence and they were both convinced, even at that stage in the 40s, that one day machines would be smarter than we are.
Graihagh - Professor Huw Price, he's Bertrand Russell Philosopher at Cambridge University...
Huw - I'm also involved with the new Leverhulme Centre for the Future of Intelligence which is focusing on the challenges of the long term future of artificial intelligence. People sometimes say, well we don't even know what intelligence is, how can we be studying the long term future of it and to that I like to say, well perhaps we should think not about what intelligence is but about what intelligence does. We know that in many ways we're the most capable creatures on this planet, and I think it's a fair bet that as artificial intelligence develops, it will be capable of doing more and more of the things that we do, and in many cases doing them faster and better. And I think we can also be certain that it will do things that we simply can't do and things that we presently can't imagine.
Graihagh - ...and this is in part why artificial intelligence is so hard to pin down. The idea is that machines would be able to do think that would normally require human intelligence. Take the game Go. It's an ancient Chinese game, with a simple concept - surround and occupy territory - but it requires a lot of abstract thinking and strategy to win but also intuition - all skills which we consider to be human. And yet Google's machine called AlphaGo beat world champion Lee Sedol 4 -1 this month. This machine, like many other intelligent machines, works by using an algorithm or a set of rules which determine what move it'll make. A bit like a training manual, so if opponent occupies this square and this square, do this. But what makes AlphaGo intelligent is that once it understood the rules, it then started to teach itself how to play better. An algorithm in itself is not all that threatening but an algorithm that can teach itself raises some red flags Huw Price again...
Huw - Nick Bostram's example is the paper clip factory which has been programmed to produce paper clips but it's smart enough to recognise that it can produce more paper clips if it removes certain sorts of constraints, eventually, it's turning the whole planet, including us, into paper clips. So it's not malicious, it's just doing what it's been programmed to do. If it discovers that it can maximise on the variables we've given it by changing one of these other ones, if it's smart enough there's not much we can do about it. That's one of the things that leads to some of the challenges we're going to face relatively soon, for example, there's a very good case for thinking that many, many jobs will be threatened over the next generation or so. If we have one of these systems making important decisions for us, in many cases it's going to be important to find out why it made the decision.

05:58 - Artificial intelligence is already here
Artificial intelligence is already here
with Professor Christian Sandvig, University of Michigan
Aritficial intelligence is already here and it's hiding in plain site. But where? Graihagh Jackson chatted to Christian Sandvig about where these secret systems are operating...
Christian - Well, I think most people think about artificial intelligence and they think maybe about some sort of sci-fi movie or something like that but, in fact, it encompasses a long of things that we'd encounter every day.
Graihagh - Christian is an associate professor at Michigan and his research looks at artificially intelligent algorithms principally in media so, Facebook and the like.
Christian - The kinds of things like recommendations for what to watch on Netflix or what you should buy on Amazon or even things like the kinds of things that your friends are doing that show up in the Facebook newsfeed.
Graihagh - How is that artificial intelligence?
Christian - One helpful thing to think about is the difference between algorithm and artificial intelligence. Sometimes we talk about how things are algorithmic but that's a really old word, it just means that there's a process or a set of steps for it. So you can see that pretty much anything with a computer is going to involve algorithms and they're going to be a set of steps because the computer needs to follow a set of steps in order to know what to do. But then there are these other cases like, for example in the Facebook newsfeed, when you sign on to Facebook and you see what your friends are doing. Here we have a system that, according Facebook's public statements, is extremely complicated and it uses many factors (by one estimate over 150 factors) to determine what it thinks that you will like. It incorporates things that you've done on the platform before like clicking "like" or commenting on other people's material and things that you typed when you posted status updates. So I think artificial intelligence is kind of lying around in plain site.
Graihagh - It's just not all that obvious, because I'm not sure I ever really thought about how Facebook tailors my newsfeed and maybe I thought it actually was an unvarnished truth of maybe a temporal relevance and organisation, but not necessarily picked and ranked.
Christian - Right, that makes sense because we have other platforms like Twitter that emphasise that it's a timeline, which is the word that they use, and so you might think that's the same for Facebook but that's not actually what happens. In fact, it's quite a complicated process to decide what to show you.
Graihagh - How they do it though is something of a mystery, people have taken a good old guess but in reality Facebook considers their intelligent algorithm valuable and so they don't share their secrets. But why do they do it in the first place?
Christian - There's a simple explanation and that's that if it's totally unfiltered, it actually doesn't work that well in some circumstances. Maybe if you've used Twitter, you've noticed that if a friend of yours is at some sort of event and they're really excited about it and they start tweeting a lot, they just flood your twitter stream with their updates and you can't see any of your other friends because they've just started posting a lot more. So there's some weird characteristics of just having everything in real time or in chronological order in the order that it was posted, that might not be ideal. Beyond that I think there's... I don't want to sound like its sinister but there's an important motive here, right. These are advertising supported companies and it's important for them to mix in advertising into your experience. They have side bar ads, but it's also very important for them to figure out ways to monetise their platform because they started out a free service, they're supported by advertising and one of the most important ways that they can do this is by mixing in ads with content and that's important for a number of reasons. One is that you can potentially get around ad blockers that way and so they have a really big incentive in being able to control your attention to the degree that they can improve the chances that you're going to look at ads.
Graihagh - Monetising aside it's interesting to hear about how there's this whole other invisible layer operating that, at least on my part, I was completely unaware of. and it's not just your timeline either, Facebook can now automatically tag your friends in a photo using facial recognition software, something that us mere mortals had to do before. In other words even Facebook is becoming increasingly automated.
Christian - It's really amazing the degree to which most media interaction today has this additional layer built into it which is a compute making decisions and because some of these decisions can be quite complicated, that means that the extra level of mediation we're not even sure exactly what the results are. Usually we implement these systems by optimising them for a certain goal like let's design a system that makes people click on this as much as possible. But these systems might optimise for all kinds of other things and we just don't understand the consequences. Unfortunately, I think we're just at the beginning of this and as we see automation spread into all kinds of aspects of our lives because computers are spreading into all kinds of access to our lives. We're going to see this as a really significant change and I don't think we're ready at all to understand the implications of that.
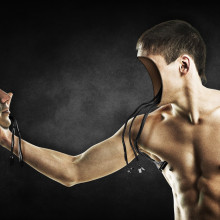
11:08 - 47% of jobs may taken by robots
47% of jobs may taken by robots
with Professor Michael Osborne, University of Oxford
Professor Michael Obsborne published a paper 2 years ago which suggested 47% of jobs are at high risk of being taken over by robots. 47% is a staggeringly high number but what jobs are safe from machines? And how will the take over happen? Graihagh Jackson put these questions to Michael...
Mike - As much as 47% of current US employment might be at high risk of automation over the next 20 years.
Graihagh - This was published a couple of years ago and there's a link to it on our website - naked scientists dot com and you know what? Michael is so well placed to talk about this, as he's not only an associate professor of machine learning at the University of Oxford but a co-director of the Oxford mountain programme of technology and employment... In other words he knows a lot about intelligent about machines and how they impact society..
Mike - So I have these two strands - these two hats that I wear. One is an academic researcher in machine learning itself, developing novel intelligent algorithms capable of processing data in an intelligent, sophisticated way. But the other hat, the second title that I mentioned before is investigating the societal impact of the development of such algorithms. So our second programme looks at the employment impact that intelligent algorithms are likely to have over the next 20 years.
Graihagh - And as you heard before, 47 % of US jobs are at HIGH risk of being taken over by robots. 47%...
Mike - It's a large figure - I should put some caveats on it, so that figure does not take into account the fact that firstly, what is actually automated would depend on regulations, societal acceptance, a whole host of other factors. It would depend on relative costs of the machines and the humans involved in those tasks and crucially we're also not taking into account in that figure the emergence of new jobs.
Graihagh - Okay then. So how did you work this number out then - this 47%?
Mike - We're drawing on data from the US from an agency called O-NET which provides for 700 different occupations, through to those occupations, a fairly long list of quantitative measures of skill requirement. So these measures would be things like a number between 0 and 100 saying just how much persuasion this required, or finger dexterity, or originality...
Graihagh - How do you determine that sort of thing?
Mike - It's an excellent question and you'd have to ask O-NET, but drawing upon that data we thought that those skill requirements might be an interesting way of determining what distinguishes an automatable job from a non-automatable job. So we cross-linked the skill requirements against the jobs that we had seen automated and against another list of jobs that we were fairly confident were not going to be automated within our horizon of 20 years. Using those two different categories and relating it to those different skill requirements, we actually taught a machine learning algorithm exactly the same kind of algorithm we're expecting might have impact on employment, the difference between automatable or non-automatable jobs and, on that basis, it was able to come back and tell us just how much of it might be susceptible to automation.
Graihagh - What makes a job susceptible to automation? There you talked about un-automatable jobs and automatable jobs.
Mike - So that was another conclusion from our study, the characteristics of jobs that are not automatable and we lumped these together into what we call bottlenecks to automation. The first of those bottlenecks was originality. Simply put, the more original your job is the less susceptible it is to automation.
Graihagh - So painters are safe?
Mike - Painters are relatively safe, yes.
Graihagh - What other skill sets did you decide were also un-automatable?
Mike - The second of our bottlenecks was social intelligence. So here we're thinking of skills like negotiation or persuasion. The kinds of high level social functions that come relatively natural to us but are relatively difficult to make explicit in such a way that those tasks could be reproduced by code.
Graihagh - And the third bottleneck...?
Mike - The third bottleneck was that of autonomous perception manipulation and this one's a little bit more subtle perhaps. It certainly is possible to get a robot to interact with physical objects in the world around it but it's important to distinguish that kind of manipulation from the manipulation that we perform in our day to day lives. So again, to give a concrete example, I'm sitting here in the studio picking up a glass of water and in doing so, I was required to distinguish the glass from the table that sits beneath it. Despite the fact the glass is transparent, I needed to, before I even picked up the glass, have some idea of how much it weighed so I could grip it with sufficient force but also have some expectation for its material characteristics so that I didn't shatter it when I picked it up by applying too much force. So you can see that, even in that relative intuitive action, I had to apply a whole host of subtle tacit knowledge about my environment that again, is very difficult to reproduce in an algorithm.
Graihagh - What would that be in terms of jobs though, because I'm not lucky enough to have someone pick up my glass of water every day and feed it to me, so where would I see that day to day?
Mike - So the kinds of jobs that might be non-automatable as a result would include hairdressing, for example, it might include gardening.
Graihagh - The bottlenecks you've been describing to me in some ways are things that are very natural to us. They don't seem to have a defined set of rules that at least I could very easily distinguish and is that, in part, why they're very hard to code for and create an algorithm for?
Mike - Exactly. We, as humans, draw upon these deep reservoirs of tacit knowledge about our society, our companions, our environment; all stuff that's very difficult to unpick and, as I say, write out explicitly in code.
Graihagh - We've talked about the jobs that are going to be relatively safe. Who is then at risk because, surely, a little bit of these skills are involved in everyone's jobs?
Mike - One particular category of jobs that's likely at high risk are those jobs that rely almost entirely upon storing, accessing and perhaps doing some simple processing of data. So I'm thinking here of examples including paralegals, whose job in large part is digging through case files. I'm thinking about people like auditors who might be required to inspect large amounts of financial data in a company. These are the kinds of tasks, to be honest, right now might be better performed by an algorithm which is able to scale to processing much larger volumes of data, is perhaps more vigilant, they're going to be just as attentive to the millionth bit of data as the first. They're not going to, for example, be influenced by how long it's been since they had lunch.
Graihagh - I suppose they don't need a sick day or anything like that?
Mike - Exactly.
Graihagh - How about radio producers and presenters?
Mike - Right. So, let me say that in this kind of social interaction that we're having, for example, there's quite likely a large amount of social intelligence that's deployed that's going to be difficult to reproduce in an algorithm so...
Graihagh - Phew. I'm safe!
Mike - Congratulations.
Graihagh - And if you want to see how safe you are, NPR have designed a nifty web tool based on Michael's work that means you can select your profession and see if you're safe or not so safe. It's on our website - naked scientists dot com or just search for will your job be done by a machine.
Mike - Firstly, a lot is going to depend on how cheap the automated resolution is relative to the human labour. Now the scary this is, of course, software has next to zero marginal cost of reproduction so once someone has developed and intelligent algorithm capable of doing a task that was previously performed by humans that software, at least in principle, can be deployed across the entire world at next to zero cost. So, in many cases, we might see relatively rapid transition to automated software solutions once that technology is developed.
Graihagh - Are you surprised or, indeed, do you think things should become increasingly automated?
Mike - On this shrewd question, of course, we shouldn't lose sight of the fact that these automated solutions are delivering products to us at increasingly low cost. On the other side of things, things are not so rosy; we shouldn't lose sight of the fact that while these jobs are replaced by an algorithm, they might not be jobs that any human fundamentally wants to do. There's an argument to be made that if a job is able to replaced by an algorithm, it's kind of below the dignity of a human being and...
Graihagh - Wow, that's pretty...
Mike - It's a strong claim I have to agree.
Graihagh - Yes...
Mike - To me, those bottlenecks that I identified before; the creativity and social intelligence are really the hallmarks of what we as human beings find satisfying and pleasurable. Consider the tasks we do in our spare time (our hobbies), they are almost universally things that involve some degree of interacting with other humans or being creative. So, to me, the kinds of jobs that will remain after automation are going to be increasingly satisfying and enjoyable
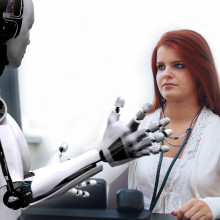
21:03 - Making a chatty robot
Making a chatty robot
with Dr Milica Gašic, University of Cambridge
Currently, personal assistant robots are not the chattiest but one scientist at Cambridge is hoping to change that... Graihagh Jackson went to meet Milica Ga?ić to find out how she's making a system that means we can have a conversation with robots...
Milica - You've probably have all heard of Siri on i-Phone or other personal systems but these systems can be more widely used ins situations like banking or they can be used for providing healthcare information for elderly people, for instance. These systems normally have three components. The first component which is called speech understanding is trying to extract the meaning from the speech. The second component which is called dial of management, tries to decide what is the best response, or what we call action, to take to say to the user and then the final component generates this response into speech.
Graihagh - None of this is trivial. Putting speech into text, understanding that and then deciding what the best action is and turning text back into speech - it's quite complicated, especially that middle step of understanding and actioning.
Currently, systems like Siri and Google all operate on a series of rules. Someone has literally sat down and thought about all the possible things you could ever want to ask your smartphone, written it into a code and - voila! Sounds painstakingly protracted...
Milica - Now this is obviously suboptimal because a human can't think of all possible situations and it's very expensive to develop such systems so, what we are doing is trying to use machine learning to tackle this problem and to make the systems better.
Graihagh - When you say machine learning, what do you mean? Are you literally sitting down a computer and saying this is X and this is Y?
Milica - Not really. The idea of machine learning is that the machine could analyse data and then try to build a model and then, based on that model, make predictions. So the prediction could be what the user wants or the prediction could be what the system should say back to the user. A particular machine learning method which is very useful for building dialogue systems is based on reinforcement learning and reinforcement learning is all about trial and error. In machine learning we normally have two ways of learning. One is supervised learning and you can think of that as you have a teacher who is teaching you. So the teacher is showing you how to do something and then what you try to do is try to imitate your teacher and do it as good as the teacher is doing it. Reinforcement learning is very different. In reinforcement learning, you explore the different possibilities. You don't have the teacher but you have say a parent who will give you occasional rewards based on what you did and if you did something well. In similar ways like children are trying to get as many presents from their parents, the system is also trying to maximise its reward and, indeed, many reinforcement algorithms were inspired from nature and how biological systems learn.
Graihagh - You just made me think of kids wanting treats. I mean is there a chocolate equivalent for a machine?
Milica - Oh absolutely! The reward is numerical and it is an equivalent of chocolate.
And speaking of food, we decided to dial up the dialog system in search of some lunch
CRIS - What kind of food would you like?
Milica - Hi, I'm looking for a Chinese restaurant in the centre please.
CRIS - HK Fusion is a nice place - it serves Chinese food.
Milica - Is it in the centre?
CRIS - HK Fusion is a nice place. Their address is 21 Burner Street city centre.
Milica - Can you tell me their phone number?
CRIS - HK Fusion is a nice place. Their phone number is 01223 355 909.
Milica - Thank you. Goodbye.
CRIS - Thank you. Goodbye.
Graihagh - You can very easily see that too and fro. That conversation it's got context of what you're asking there. So, from that conversation it's learning every time someone dials and asked for a specific food (whether that's Chinese or pizza), but what will it be learning from that conversation or how will it be learning from that conversation?
Milica - So, from time to time it would be asking somebody to enter their feedback. At other times, it's trying to estimate feedback on its own and then what it does is it tries to analyse which actions it took and what feedback it got. One thing it wants to maximise is the chance of success. When it provides all the information that the user's asked for it's counted as a successful dialogue but that is not the only component it is trying to optimise. It also tries to offer as much information as possible in as few turns as possible because users generally don't like to hang around and talk to dialogue systems forever. So it tries to adjust its actions so that it optimises these two objectives.
Graihagh - So it sort of almost goes away and reflects? Not unlike a human; what was good and what was bad about that conversation.
Milica - Yes exactly, that's a very good comparison.
Graihagh - So in the future do you envisage this being much more broader than just ordering Chinese food in the city centre.
Milica - Yes. My goal is to model a more richer conversation. In particular, one idea that I have is to build a dialogue system that can be used for the prevention of mental health illnesses and the idea would be to develop a dialogue system that everybody could access on their phone, whenever they like, whenever they have a problem they could get anonymous instant support. So I think that would certainly have a huge impact but also from a scientific point of view, these dialogues would be much richer so it wouldn't be about ordering Chinese food but rather about trying to model real conversation.
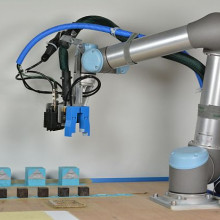
28:17 - Mimicking evolution
Mimicking evolution
with Georgia Mills, Naked Scientists
If a robot is going to take over the world, we've got to get to a more general artificial intelligence where a machine can think for itself. So, how do we do that? There are two ways and both are inspired by nature itself. One is by reverse engineering the brain and the other is by copying evolution. Graihagh Jackson sat down with Georgia Mills to see how this would work in practice...
Georgia - We're going to use evolution to make the next generation of super robots.
Graihagh - So if we take us humans as examples, how did we get clever?
Georgia - Well, there are a number of different theories on exactly when and how humans and our ancestors became intelligent and, even though we don't know the exact reason we became smart,
we do know the mechanism and that's evolution. So, the individuals who weren't so smart gradually got booted from the gene pool, essentially...
Graihagh - To put it nicely...
Georgia - Yes. Booted - dead. Meaning that as a species we got smarter and smarter and then, eventually, we get to where we are today where we are able to cure diseases, sending rockets into space...
Graihagh - I mean this is a really complicated process; it involves genes and environmental pressures. So how do you mimic this?
Georgia - Well, if we think about evolution it involves a code, so that's DNA, and when a living thing has offspring, there are tiny changes in this code which is maybe caused by mixing the DNA with another organism or by errors in the code, so that's a mutation and this causes variation. And if some things do better than others in this variation, we have what we call natural selection which is kind of the bare bones of evolution. So if you take this idea and change it from a biological code into computer code...
Graihagh - Simple!
Georgia - Simple! You can create a system where these little iterations crop up in each generation of code and then you can have a system in place, which acts as the natural selection, testing the code for the output you want. It selects the ones that are most successful and then the idea is, as each generation goes on, you get better, and better, and better at doing what you want to do.
Graihagh - All I'm imagining is robots having sex and I'm assuming that's not quite how it's going to work in practice...
Georgia - Maybe one day!
Graihagh - I'm not sure I'd want to witness that though.
Georgia - I would.
Graihagh - That is recording Georgia. You do realise everything I record is licensed to go in?
Georgia - You're not going to use that... So at the moment it's more like the idea of an algorithm that makes this little piece of software run around on a computer screen - that kind of very basic thing. But there is an example here in Cambridge where someone has built a robot that can make these little babies, test them and then make more and more babies that can go on and do better and better. I actually went to see this robot at a conference and I was introduced to it by a Louis Osprobreck. It looks to me like one of the stereotypical factory robots - a sort of overreaching arm. What is it actually trying to do?
Louis - It is a factory robot. It has a gripper and like a hot glue gun in front (an industrial one). So it only has two types of components; it has wooden cubes and he has those cubes with the motors in there and it glues them together to build the robot. We are trying to automate a design for a robot so it's randomly coming up for a robot that walks and we are iterating this design by testing it. So we built ten robots and we test how it works and then take the best ones and use like evolution to generate a next generation of robots. And then we built them again, test them again, and so on. And eventually the small robots can move forward when we turn on its motors. Over there, that's one of the working robots.
Georgia - Can I see it walk?
Louis - Sure...
Georgia - I don't know quite how to explain what just happened. The squares sort of had a spasm
and then it moved around. What's going on here?
Louis - As I say, we glue these cubes together and two of them have a motor in there which can move one side of the cube back and forth. We don't know in advance whether it's going to work and how it's going to work, but somehow it moves away.
Graihagh - They were doing what! They were crawling?
Georgia - Crawling is a kind way of putting it. Imagine two cubes just stuck together and sort of swizzling about and then, somehow, this kind of cube abomination sort of shuffles slowly across a table.
Graihagh - Sounds great, but what is this demonstrating.
Georgia - So this cube whizzing along is, actually, twice as good as the first cube this robot created but what was interesting, there was no input from a coder or an engineer to make this new one better - it was all left to the robot. So what happened is it made ten little babies and in these baby cubes there are these variations in quite how they're glued together, and then these ten babies are tested against each other and the fastest one of these cubes that twists along it's sort of said, you're the winner, you've survived, you're in the gene pool. The computer takes the design from that cube baby and says, this is the winner. Makes ten slight iterations from that design, like the mutations I mentioned earlier, and then does the whole process again. So the idea being each generation the designs get slightly, slightly, better and I think after ten generations they got twice as fast at crawling.
Graihagh - I imagine you'd be able to scale this up beyond just crawling into other skills that we might actually need to form something that resembles artificial intelligence then?
Georgia - So there are examples of people using this kind of mimicking of evolution to design the best kind of antennae or to design mirrors that can funnel light for solar panels, and things like that and there's no reason, in principle, that it can't be used to try and make intelligent computers.
Graihagh - In a way then these computers are thinking outside the box. They're thinking in ways that humans wouldn't be able to think unless thinking of ways that they can change and evolve to be much better in their design than perhaps we even could design them to be.
Georgia - Well, I'd hold you back on the word thinking there. There is no thinking going on. It is just making random changes in this code and then, by just simply testing and changing, and testing and changing again, the actual effect we get is something that looks like this robot has been really creative and has solved the problem but really, it's just using this random process of evolution.
Graihagh - By having this process being random, I'm assuming that's not perfect. There aren't environmental pressures which say, do this, it's completely random, so surely there's got to be a catch here.
Georgia - You've got your finger on it right there. So the thing about evolution; it doesn't say let's go and have great big brains, that's exactly what we need. There are a whole host of things that might be selected for. There's also the problem that evolution, unfortunately, takes millions of years so we want to speed the process up. And the other problem is mutations - I mentioned them earlier -they're often bad. If you think about mutations, they're associated with things like cancer, but we have the advantage here so we can be a bit sneaky. We can say only good mutations in the code from now on, we can speed up generation times and things like that, and we can say we only want it to be selected for intelligence. So we have these advantages here over evolution, but it's still not clear if it will actually be enough to get these super-intelligent computers.
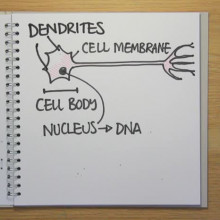
36:33 - How one man is copying the brain
How one man is copying the brain
with Dr Richard Turner, University of Cambridge
Another way to create artificial intelligence is to copy the human brain. But it'll have to be done in stages. Step one is to copy how our senses made sense of the world and Richard Turner is focusing on hearing. This has proven tricky though, as he explained to Graihagh Jackson...
Richard - Well simply put the brain is the most complicated that we know about in the whole universe at the moment. It contains, roughly speaking, 100 billion neurons which are wired in an incredibly complicated way and it's able to use that structure to process incoming information, make decisions about what it should do in light of that information action those decisions i.e. make your muscles move. At the moment, we know next to nothing about how that entire system carries out those three basic, fundamental operations, although by looking at particular parts of the system where we think we know what the functionality is, we are able to make some small progress.
Graihagh - When you say some small progress, you mean in copying or what you might call reverse engineering the brain?
Richard - Both. So I've been looking at the principles by which people process sounds and can they be used to developed computer systems for processing sounds in the same ways that people do.
Graihagh - How we process sound is remarkable. Right now, if you stopped what you're doing, how many different sounds can you hear? 3? 4? 5? 10? Yet, you can still listen to me and not be distracted by all those sounds. Now, think about it from a machine's perspective. How does it know which sounds to ignore and which ones to pay attention to? Well, Richard's got that sorted. by understanding the statistics of a sound, you can make a machine learn to distinguish different noises from a camping trip say...
Richard - In the clip I start by a campfire and then you'll hear my footsteps as I walk through gravel. Then I go past a babbling stream and the wind then starts to get stronger, and I unzip my tent and get in and it turns out I do this just in time because it starts to rain...
Now all of those sounds are examples of what we call audio textures. They're comprised of many independent events like individual raindrops falling on a surface which in combination we hear as the sound of rain. Perhaps what's remarkable about those clips is each one of those sound is, in fact, synthetic. It was produced by taking a short clip from a camping trip and then training a machine learning algorithm to learn the statistics of those sounds.
Graihagh - I mean, I think of a sound as sound; I don't see it or hear it as a series of statistic. So what do you mean by that?
Richard - Take the rain sound, for example. The rain sound when you take short clips of it contains different patterns of falling raindrops and so the thing that is constant through time is not the soundwave form itself, its the statistics; its the rate which these raindrops appear and the properties of the surface and so on and so forth.
Graihagh - Okay I see. So this computer has emulated those sounds - it's a synthetic sound. Why is that beneficial in helping you reverse engineer the brain?
Richard - Yes, that's a good question. At the moment this just sounds like a cute trick so...
Graihagh - I was going to say I'm loving it from a radio point of view. I could use that sort of computer in my line of work...
Richard - Indeed. But when we look at the way the computer algorithm does this, we think that it's using principles which are similar to those which are used by the human brain.
Graihagh - When you say we think, it makes me think you're unsure. Does that mean you're not entirely sure how the machine is working out or the other way round, you're not sure how humans are able to distinguish and refocus their ears?
Richard - We're unsure in the sense that we don't know whether the brain in the machine is operating in the same way that the brain of a person is, even thought it responds in a similar way.
Graihagh - But hand on a second. You've built this machine, how can you not know how it works?
Richard - Well this is one of the beauties of machine learning. I've been looking at are what's called unsupervised algorithms. So you just get data, the data is unstructured, you don't know what's going on, you don't even knows what's good to predict and so the algorithm itself has to figure out what the structure is. So, I think much of the future advances in machine learning will move towards these unsupervised settings and why I think they're really interesting is that's the setting that the human brain is faced with. It's not given a teacher which is able to give it the labels of millions and millions of objects, it has to automatically adapt (maybe it gets a few labels from your mum and dad), but the number of labeled examples you get is essentially zero when you compare it to what our current state of the art object recognition system is given.
Graihagh - So, in an ideal world, we'd scale this up. This is obviously just looking at sound but we'd scale this up to emulate what - the whole brain and in everything that we do?
Richard - To put it in simple terms, we have no idea of the software that the brain runs at the moment and it's going to take a long time to figure out details of that software that would be necessary to come up with, say, super-intelligent algorithms.

41:54 - Will robots take over the world?
Will robots take over the world?
with Martin Robbins, Guardian Science Writer
There may be a long way to go when it comes to figuring out the details of creating super intelligent, humanoid robots - in principle though, it's possible so let's say, hypothetically, we were able to make an a super intelligent algorithm. How would we get from something that operates from a hard drive to the likes of Skynet? Kat Arney spoke to Martin Robbins to find out whether something like this is even possible...
Martin - No!... Or if you want the long answer... No!
Graihagh - Well, that's shut me up hasn't it...
Martin - My name's Martin Robbins. I've worked in A.I. in industry for the last eight or nine years and I write the Guardian's "Raising Hal" blog on artificial intelligence and data science.
Graihagh - Martin and our Kat Arney sat down for a cuppa to mull over why the terminator isn't going to become a reality any time soon...
Martin - The idea of the singularity has been around in one form or another since the 1950's and it's become more popular in the last 20 or 30 years. The idea behind it is that at a certain point of development, an A.I. would become better at producing successive versions of itself than a human would. Once that happens, then it's evolution would become, essentially, unpredictable and, essentially, the singularity is the point at which we have no idea what would happen.
Kat - Do you actually see that happening in the future?
Martin - I think it's a question that's almost impossible to answer. In a way it's very similar to the Victorians speaking what constitutes life and how do we create life. So, if you go back 120 years, people like Mary Shelley were obsessed with the idea that you could reanimate a corpse and somehow take a inanimate object and imbue in it with the qualities of life. The problem with that is you immediately start falling into all these semantic questions and practical questions about what do you mean by life. What does it mean to give life to an object and you hit the exact same problems when you start talking about sentience. Until you've got a meaningful working definition of what it means to be sentient that comes beyond just philosophy and, actually, has some kind of practical, real world equivalents. There's just no real way to answer the question.
Kat - I guess one of the problems is when we see depictions of artificial intelligence, robots for want of a better word, in films, there's this idea that they have feelings, thoughts, they have interactions with humans. Is this just us projecting onto A.I.s what we think that they might be - building them in our own image in our own minds?
Martin - Well that's part of the problem. Every time we talk about something like sentients, we immediately start to bring to that word all the baggage that we have from our own experiences of being a sentient creature. So we talk about artificial intelligence and remember that artificial intelligence at its root is purely about trying to recreate mechanisms of intelligence and problem solving that humans use. As soon as you start talking about motivation, or emotions, or feelings, or goals, or objectives, all of these other things, you're going beyond the original remit and you're starting to link intelligence to lots of things that are basically part of the human experience and this is one of the problems really. If you look at the way we relate to artificial intelligence, it's incredibly ego driven and it becomes less about what would an intelligent system be like and it becomes more about how we create something that we can relate to and that sort of reproduces us in an immortal or god-like form.
Kat - Is there any inkling that an artificial intelligence would like humans, hate humans, want to destroy us?
Martin - You've literally no idea what direction this could go in. It might want global conquest or it might be happy like trolling chess programmes. There's no reason to think it's going to do one thing or the other.
Kat - Particularly in films or in popular culture, there's this idea that intelligent robots will rise. The idea of a clone army or robotic artificial intelligences taking over but I've seen videos robots trying to walk, trying to do stuff. I think there's quite a long way to go here - am I right?
Martin - Yes, I'd say so. There's obviously been a lot of interest recently in companies like Boston Dynamics who've been experimenting with robots that walk on legs. At the moment, the state of walking robots is pretty poor. They can go a short distance but they need a lot of supervision. There's no way that you take a robot and send it off across several miles of open terrain and expect it to get back again in one piece unless it was on wheels and the terrain was reasonably flat.
Kat - So no marauding armies of robots anytime soon?
Martin - No - not soon anyway.

46:55 - What about the robotics?
What about the robotics?
with Dr Philip Garsed and Dr Rachel Garsed
Scientists have made huge advances in robotics. Prime examples are key hole surgey and the self checkouts in supermarkets. But when it comes to making automous robots that can walk about, it's proving to be rather tricky. Graihagh Jackson went to dinner with Philip and Rachel Garsed to find out why...
Phillip - I'm Philip Garsed and I design electronics for large physics experiments.
Rachel - I'm Rachel Garsed and I'm an electrical engineer at Cambridge Medical Robotics.
Graihagh - And of course, what comes after dinner? Cupcakes! Except, they weren't decorated and during the main course, Rachel conveniently turned into a robot to show me just how difficult it is to programme a cyborg to do a simple task, yet alone become entirely autonomous in the larger world.
Philip - We've got here Rachel, our friendly robot and what we're going to do is programme her and it's a bit like programming a computer but, in this case, she's going to do some physical tasks and Rachel knows a few commands that we can give her. So firstly, she knows about things, objects...
Rachel - I know the difference between sprinkles, cake and buttons.
Philip - We have to construct a programme from the different things that the robot understands to do the right sequence of things to get what we want to happen.
Graihagh - So we give it a series of commands?
Philip - Exactly. It's almost like having your own recipe. You have to do everything in the right order otherwise you get some horrible disaster.
Graihagh - So what commands do you understand, Rachel?
Rachel - I understand pick up, put down, unload and rotate. Oh, and also stop.
Graihagh - Okay, let's take it way. Let's see how I do - I'm feeling pretty confident.
Philip - Okay....
Graihagh - You've made me (Blue Peter Style), here's one you made earlier. So it's got four chocolate buttons on, a series of sprinkles and icing and that's what I'm going for.
Graihagh - Pick up sprinkles...
Philip - So that worked quite well. She's holding the sprinkles...
Graihagh - Unload sprinkles onto cake... Stop, stop, stop, stop!
Philip - That didn't quite go to plan did it? So you've stopped the robot but, currently, there are sprinkles absolutely everywhere. So what do you think went wrong there?
Graihagh - I didn't specify how long to unload for...?
Philip - Exactly. We have no way of telling the robot, at this stage, when to stop. It doesn't know how many sprinkles are too many. What we can do is specify a time, I'll get another cake...
Graihagh - I not we we're going to have any sprinkles left by the end of this...
Rachel - We've bought extra - we've brought more...
Graihagh - Okay so we're going to start again from the beginning. Pick up sprinkles... Unload onto cake for one second... Put down...
Philip - Job one complete.
Graihagh - Better?
Philip - That's better but did you see how unexpectedly it went wrong and you didn't even have a clue that it was about to go wrong.
Graihagh - No, I suppose it's just that common sense in me that would expect to not to pour that many sprinkles.
Philip - Robots have no common sense - we have to programme it.
Graihagh - Pick up buttons... Oh...
Philip - Right - you've got about a hundred there. Is that as many as you wanted?
Graihagh - I need four but probably one at a time I should imagine. Put down - Rachel... Pick up one button... Unload on cake... Winning...
Philip - Right, exactly. But do you remember before how we we able to tell the robot to do something for a certain amount of time or for a certain condition? You'll notice here that we're doing the same thing many, many times so this is actually where robots start to come into their own. Because we can say for four buttons - pick up one button, unload button onto cake, rotate cake and because it then knows that that's got to happen four times, it will then do it for button number two, button number three, and button number four.
Graihagh - I went wrong at every single instruction, pretty much. I poured sprinkles everywhere, I stacked chocolate buttons on top of each other and this is still what I would consider a really simple task...
Philip - And yet, Rachel pretending to be a robot is about as sophisticated as we could get because we're really still using her human intelligence. She knows how to pick up a tube of sprinkles without crushing it and all these little things, she can identify exactly where the cake really is and, in reality, robots are much more limited than that. It shows you how versatile humans are and really how much you have to limit the problem if you're trying to interact a robot with the outside world.

52:06 - Should we take AI seriously?
Should we take AI seriously?
with Professor Huw Price, University of Cambridge
Artifcial intelligence is already here and it's only going to get smarter and more ubiquitous. They'll take our jobs but could also enhance our lives beyond belief. Given that, should we be taking this revolution more seriously now? Huw Price thinks to and he explained why to Graihagh Jackson...
Huw - There's a good case for thinking that probably over the course of this century, we're going to be facing one of the biggest transitions that our species has ever gone through. Whatever it's going to mean, it's a challenge, and whatever its downsides and upsides, they're things that we face together and I think it's very important to recognise that fact.
Graihagh - And to recognise those facts, we have to know the risks. Like how even if we do lose our jobs to robots, how we then perceive ourselves...Will we have a crisis of identity?
Huw - There's a sense in which each of us has many different identities as members of different sorts of communities. Work communities and family communities and it may be that we need to shift things a little bit away from the kind of work-focus into finding what we are, and make some of the other kind of focuses more important.
Graihagh - It's an interesting point you make, because I definitely define myself, in parts certainly, by what I do - I make radio. I mean, what are we without our jobs? I'm not sure how I would define myself. Hi I'm Graihagh - I'm a radio producer. I mean I don't know what else I'd say.
Huw - Well, perhaps you say that when you're meeting people to interview them but it may be that in another context you introduce yourself in other ways. I mean, certainly if I'm in a taxi and the taxi driver starts chatting, I'm very careful not to say I'm a philosopher and partly that's because taxi drivers tend to have their own philosophies and be very keen to tell you about them.
Graihagh - So how do you introduce yourself then?
Huw - Well sometimes I say, well I'm a physicist.
Graihagh - What, because you won't get any questions?
Huw - Exactly. Taxi drivers don't have their own physics...
Graihagh - Okay - learned lesson but still you're saying a physicists. That's still is work-related.
Huw - That's true and, as I was saying, I do think that to some extent, we've got to move away from that. I think some people will end up having a more what you might call, multidimensional kind of work life but also they'll have more time for other things.
Graihagh - Yes, and it's interesting you say that because something that came up with my interview with Mike was that actually, algorithms and machine learning may free us up to do things that are much more enjoyable in life. You know there may be the cons that we lose jobs on the way but it might mean we have a much more satisfying job.
Huw - Yes, exactly. I think there is a wonderful opportunity here, but it's the kinds of changes that are needed to make the best of it are probably quite far-reaching.
Graihagh - We've talked a little bit there about what the benefits could be in terms of better jobs and stuff but I'm wondering how you might have a more satisfying job but actually, how machine learning might seriously enhance what we do. I'm thinking medical images when you're looking for metastases of cancer or whatever, a machine that could endlessly and tirelessly look at those images and work out which were needed for further screening or not. I could imagine something like that having real benefits to not only that individual doctor, but also society as a whole.
Huw - Yes, and I think there'd be many cases like that where machines will soon be better at doing those sorts of tasks than we are. Fortunately, there's also a growing sense that the community of people involved are increasingly aware of, as it were, how much of our future they hold in their hands and there's a very encouraging sense of a growing community coming together within the tech community as well as from the outside - from academics - to get together to meet the kinds of challenges of the area and trying to ensure that we maximise the potential benefits.
Graihagh - So when you look to the future of A.I., what do you envisage our future to look like?
Huw - It's very hard to do this long term prediction but I think I am an optimist. I think there are, of course, risks we need to consider but there's a huge potential for it turning out to be something rather wonderful. The example I'm going to give you is a little bit contradictory but imagine that before the development of sophisticated language, ancestors were trying to think about the question as to what they might be able to do if they had language. It's obvious that they wouldn't be able to get very far and yet what was ahead of them, in many ways, was something quite remarkable, and I'm optimistic that that's the case with A.I. too. We don't know exactly where it's going but we do know that some of the possible destinations are really wonderful ones. We want to work together to make sure we get to the good ones and not to the bad ones.
Graihagh - Huw's right - Artificial Intelligence could be revolutionary but we're future gazing here - it's all a guestimate - and thus it's hard to know what our future really holds.
So after ruminating and chewing overall the possibilities what can we take away from this?
That AI is already here...
Christian Sandvig - In fact, it already encpasses things a lot of things that we'd encounter every day.
Graihagh - ...and you know what, it's only going to get smarter. Yes, it may take some of our jobs...
Machine - What kind of food would you like?
Graihagh - ...but actually, like all technological revolutions, it will give a lot back too.
Michael Osborne - The kinds of jobs that remain after automation are going to be increasingly satisfying and enjoyable.
Graihagh - Advances in technology have also saved us time, money and given us home comforts (I cannot for one minute imagine life without a washing!) I'm sure artificial intelligence will do the same in ways we can't even imagine.
Huw Price - I think I am an optimist, I think of course there are risks that we need to consider, but there's a huge potential for it turning out to be something rather wonderful.
Graihagh - As we hand over more jobs to machines, it will have some very real human consequences - new questions, not just about our identity, and expose flaws and challenge us. But that's exciting!
For now, we've only just scratched the surface when it comes machine learning, smart algorithms and robotics... What I'm trying to say is that I'm not going to worry about computers taking over the world any time soon and you shouldn't either, but it might be worth checking if they'll take your job first!
Comments
Add a comment